I’m constantly reflecting on what this latest wave of AI means for the companies of tomorrow.
While it’s dangerous to fall victim to the kind of hubris that makes you think that you can predict the future, it’s still useful (and pretty interesting) to try and play things forward.
Something I’ve been reflecting on is what generative AI means for new businesses outside of the use cases we see today.
It seems to me that Generative AI has become a bit of a misnomer, at least in the business world. Understanding why is necessary for understanding what can come next.
Because of the name and the most popular early use cases - writing copy, creating images, populating data tables - people think that generative AI is about generating some kind of content.
And in fairness, almost all business use cases to date suggest as much. But it’s important to consider that the “generative” in generative AI is not about content creation and is instead about the underlying scientific approach.
Why is this so important to understand? Bear with me.
I spoke to Alex Long, an Applied Scientist at Amazon about this, and he said:
'Generative models' used to refer to models primarily concerned with capturing the underlying probability distribution, which made them versatile for various tasks. It was a broader term than generative in the sense that you create images or text or audio or whatever. But the reality was they were not as effective as discriminative models for common tasks like classification until the recent advancements which started with GANs in 2014 and has taken a huge leap forward in this latest wave.
Check out this two minute video (from eight years ago!) that explains the difference.
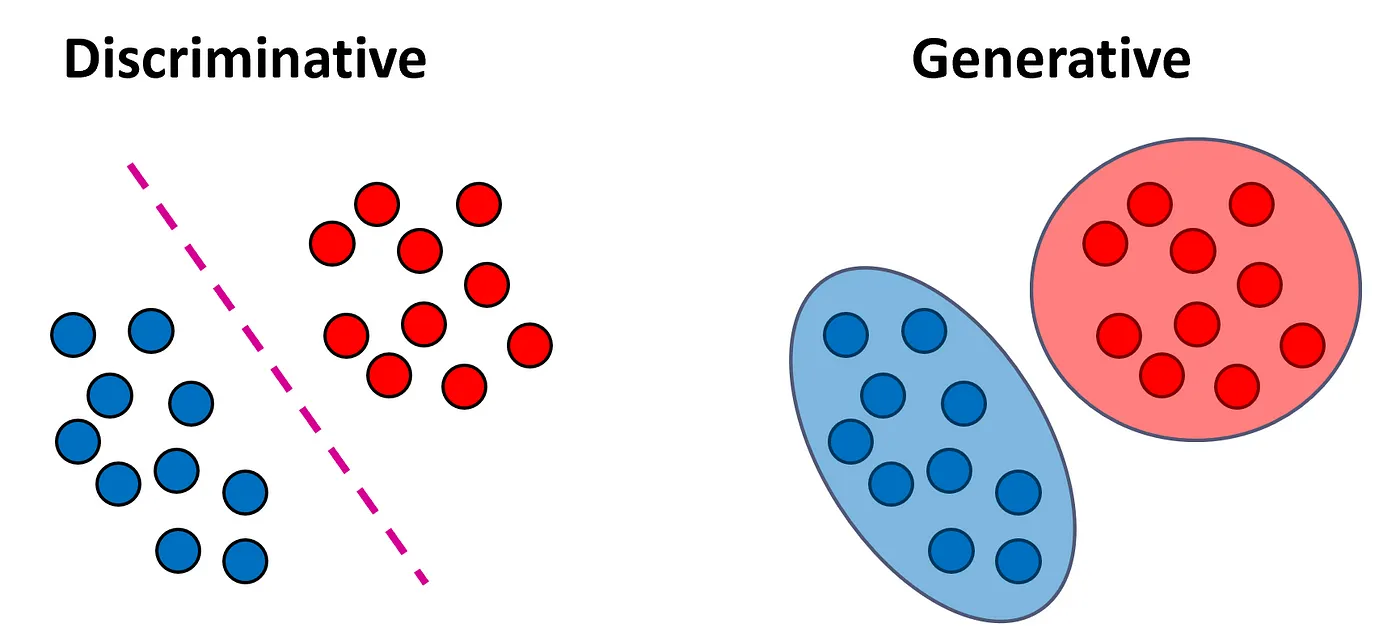
What does this mean for businesses?
A lot of the business use cases and startup ideas I’ve seen today have been heavily grounded in the idea that we can use this technology to create words or images. People mostly draw the line there.
But as industry begins to build applications beyond content creation, we’ll start to see a broader set of even more useful solutions to everyday problems. That is what excites me as an investor.
In the words of Elad Gil, from his most recent blog post:
When many business people talk about “AI” today, they treat it as a continuum with past capabilities of the CNN/RNN/GAN world. In reality it is a step function in new capabilities and products enabled, and marks the dawn of a new era of tech. It is almost like cars existed, and someone invented an airplane and said “an airplane is just another kind of car - but with wings” - instead of mentioning all the new use cases and impact to travel, logistics, defense, and other areas.
I asked a different researcher to the above for examples that come to mind outside of what people in the business world see as “gen AI”. They said:
- Leveraging reasoning/chain-of-thought capabilities of verbal models. Most product-first LLM startups are doing some version of this, I guess.
- Building on that, ML explainability could be richer than it has been? I've only seen people using reasoning behind the scenes so far, but maybe somebody will figure out how this could be cool for users.
- Recommendations: this is being tackled from many possible angles, from very wordy "conv-rec" to people leveraging the more abstract sequence-to-sequence ability of transformers. I'm yet to see the results be strikingly good, but there are glimmers of interest and it's certainly different from the boring old latent-factor approach.
- Information retrieval: there was a paper a while ago on getting transformers to memorise hashes instead of doing indexing. It's a cool idea but I'm not sure if anybody's gotten it to work in production.
- Again on information retrieval, using an LLM as an intermediating layer between a user's vague/broad intention and a large datastore that is optimised to answer specific/narrow queries looks like it has a lot of potential as far as I can tell.
Others said that there’s a research team using generative AI for robotics and another said that it has potential to help identify new molecules with specific properties for drug design.
My overarching point in sharing the above is that I think there’s a lot yet to come from this step-change in AI capability beyond language/image generation and that the applications could be much broader than many of us expect.
I’m hoping this will coincide with true enterprise adoption, which should drive a virtuous cycle of AI adoption and business creation. Many enterprises today seem at a loss for how to incorporate this latest wave of AI into their operations and products, but I expect that a wave of startups allowing for new use cases and more mindful enterprise security will help enterprises come up the curve.
If you’re founding a business in the AI space, we’d love to hear from you. Get in touch at casey@squarepeg.vc. I’m also happy to simply hear your feedback.
If you’d like to hear more about our thoughts on AI at Square Peg, subscribe to this blog and my newsletter, Artificially Intelligent.